In recent years, commercial and industrial facilities have seen a surge in the adoption of analytics packages and artificial intelligence (AI) solutions for HVAC system optimization. These technologies promise to revolutionize how we manage and optimize our heating, ventilation, and air conditioning systems. However, reality often falls short of these lofty expectations, particularly when it comes to complex, dynamic systems like chilled water plants. This blog post will explore the limitations of analytics-only and machine learning approaches and discuss why complete optimization requires a combination of smart analysis and automated action.
Defining Chiller Plant Optimization
Pretend your chiller plant is a sports car. Optimization is akin to fine-tuning a high-performance sports car. Just having a powerful engine and the latest dashboard sensors isn’t enough – you need to continuously adjust the fuel mixture, gear ratios, suspension settings, and dozens of other parameters to extract maximum speed and efficiency from the vehicle. You can’t do these things while driving, you can’t do them quickly enough and correcting one parameter may inadvertently upset another.
Optimization is the process of automatically and continuously monitoring the car’s performance, identifying areas for improvement, and automatically making the right adjustments at the precise moment the adjustment is needed. Without this optimization, the car would never reach its full potential, no matter how impressive the underlying technology.
The same principles apply to chiller plant optimization. These complex HVAC systems have numerous interrelated components – chillers, cooling towers, pumps, valves, and more – that require continuous fine-tuning to operate at peak efficiency. Analytics and AI can provide valuable insights into system performance, but optimization goes beyond just monitoring the data. Complete chiller plant optimization uses real-time, adaptive algorithms to automatically adjust control parameters in real-time, minimizing energy consumption and operating costs without the need for manual intervention. Just as in a high-performance car, the key to unlocking maximum efficiency in a chilled water plant lies in the optimization process, not just the underlying technology.
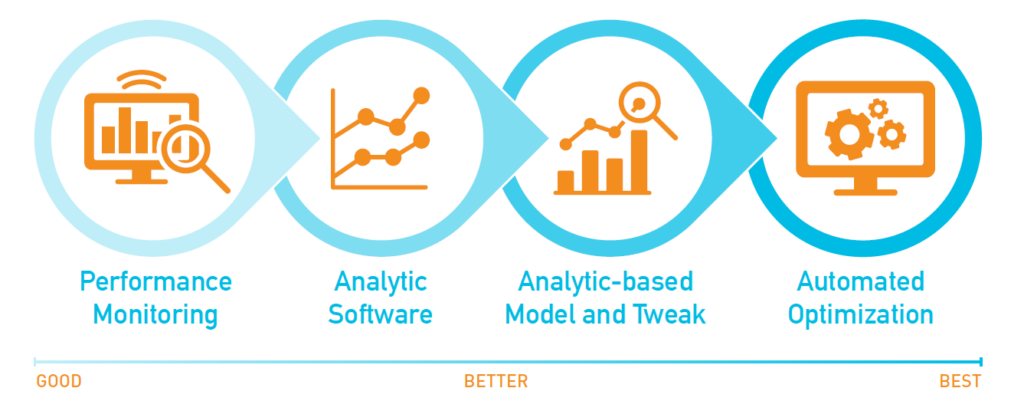
The Limitations of Analytics and AI in Chiller Plant Optimization
While analytics and artificial intelligence (AI) have made significant strides in HVAC system optimization, their application to chiller plant optimization reveals several limitations that are important to understand:
1. Passive nature of analytics and AI:
Analytics tools and AI models can identify issues, predict potential problems, and suggest improvements. However, they cannot implement changes on their own. This passive nature means that even the most sophisticated AI-driven analytics may fail to improve system performance if actions aren’t taken based on their insights.
2. Reliance on human intervention:
Despite advances in AI, most systems still require human oversight and decision-making. This dependency on human intervention can lead to delays in implementing optimizations, especially in facilities with limited staff or expertise. Even when AI suggests actions, a human often needs to review and approve these suggestions before implementation.
3. Complexity of dynamic systems:
Chilled water plants are highly dynamic, with numerous interrelated variables that change constantly. While AI can process complex data patterns, the sheer number of variables and their rapid changes can sometimes overwhelm even advanced AI models. This complexity can lead to suboptimal recommendations or missed optimization opportunities.
4. Time lag between insight and action:
In a fast-changing system like a chilled water plant, the delay between receiving an AI-generated insight and implementing a change can result in missed optimization opportunities. By the time a human is able to review and implement an AI recommendation, the optimal conditions may have already changed.
5. Data quality and quantity dependencies:
Both analytics and AI systems are only as good as the data they receive. Poor data quality, insufficient historical data, or gaps in real-time data collection can lead to inaccurate insights or predictions, regardless of how advanced the AI algorithm might be.
6. Lack of contextual understanding:
While AI can process vast amounts of data, it may struggle with contextual factors that aren’t easily quantifiable. For example, an AI platform may not account for scheduled maintenance, upcoming weather events, or changes in building occupancy patterns unless explicitly programmed to do so.
7. Over-reliance on historical data:
Many AI models heavily rely on historical data to make predictions and suggestions. While this approach can be effective, it may struggle to adapt to unprecedented situations or fail to identify novel optimization strategies that haven’t been tried before.
These limitations underscore a crucial point: while analytics and AI provide valuable insights, they are not standalone solutions for HVAC system optimization. The passive nature of these technologies, coupled with the dynamic complexity of chilled water plants, creates a gap between information and action. This gap can only be bridged through a more comprehensive approach that combines analytics and AI with automated, responsive control mechanisms.
Automated Optimization and Chiller Plant Optimization
Automated, real-time optimization uses advanced control systems that continuously adjust the operation of various components in the chilled water system to maximize efficiency and performance without human intervention.
Unlike traditional control systems that rely on fixed setpoints or simple schedules, these adaptive algorithms dynamically adjust parameters such as chiller sequencing, pump speeds, and cooling tower operations in response to ever-changing conditions. The real-time nature of this optimization ensures that the system always operates at peak efficiency, regardless of fluctuations in cooling load, ambient conditions, or equipment performance. Moreover, these systems take a holistic approach, considering the complex interplay between all components to optimize the entire plant as a unified system.
Chilled water plants exemplify the type of dynamic system that benefits most from automated optimization. Here’s why:
- Constant fluctuations: Building cooling needs can vary dramatically throughout the day based on occupancy, equipment usage, and activities. The efficiency of chillers, cooling towers, and pumps can fluctuate based on operational conditions and wear.
- Complex interactions: Chilled water systems are characterized by intricate interdependencies. Adjusting one parameter, such as chilled water temperature, can have cascading effects on pump speeds, cooling tower performance, and overall energy consumption. Many of these interactions are non-linear, making it difficult to predict the outcome of changes without sophisticated modeling. Optimizing for energy efficiency while maintaining comfort levels and equipment longevity requires balancing competing priorities
- Speed of response: Conditions that affect efficiency can change in seconds or minutes, requiring near-instantaneous responses. Anticipating and preemptively adjusting for upcoming changes (like a sudden increase in cooling load) can significantly improve efficiency.
Analytics and AI are powerful tools, but they are just pieces of the optimization puzzle. To truly implement chiller plant optimization, facility personnel must look beyond analytics and AI alone and embrace solutions that can translate insights into immediate, automated actions.